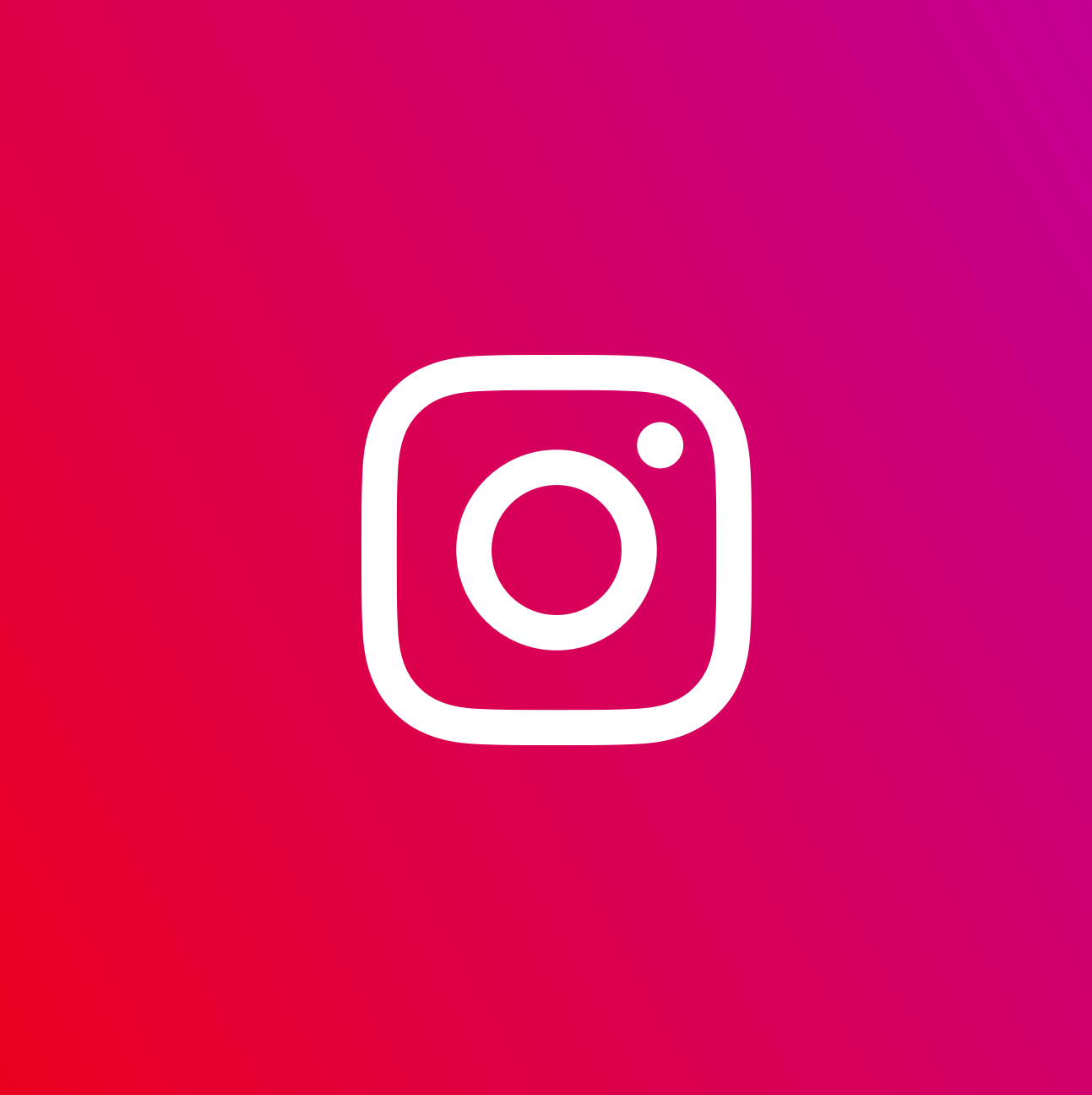
Recommending Reels content quickly and reliably for millions of users.
I joined the Reels Recommendation team at Instagram shortly after graduating college. It was an amazing first job. I found myself thrust into a world where millions of users are a drop in the bucket, and downtime means tangible, serious consequences. Instagram is truly one of the few places where one can learn at this scale.
As part of my work on the team, I had to learn a lot about reliability, and how to make systems work with such monumental amounts of data being gathered and generated. I had to process millions of videos and audio clips every day, and make sure that the systems I built could handle the load.
I built a number of systems used in the Reels recommendation pipeline, including a pipeline used to categorize short-form videos using video and audio machine learning models. This was then used in the recommendation system to help serve the user diverse and interesting new content.
Another highlight was being able to contribute to offline ranking, which ranks pages of Reels when the user is not in app, at cheaper, off-peak computation times. This helped keep the experience snappy for users, with freshly recommended content, while also saving resources when they were most needed (at peak times). This was interesting in that it provided a look at how to save money and resources in a very tangible way, while still improving the user experience.
I ultimately left Instagram to pursue General Task. Instagram was (and still is) an amazing company, and Iām very grateful for the opportunity to have worked there. I learned a lot, was able to work with some of the smartest people in the industry, and was able to ship contributions that millions of people use every day.